Summary of the key AI models and technologies
- ArborESG Tech Research
- Sep 21, 2024
- 8 min read
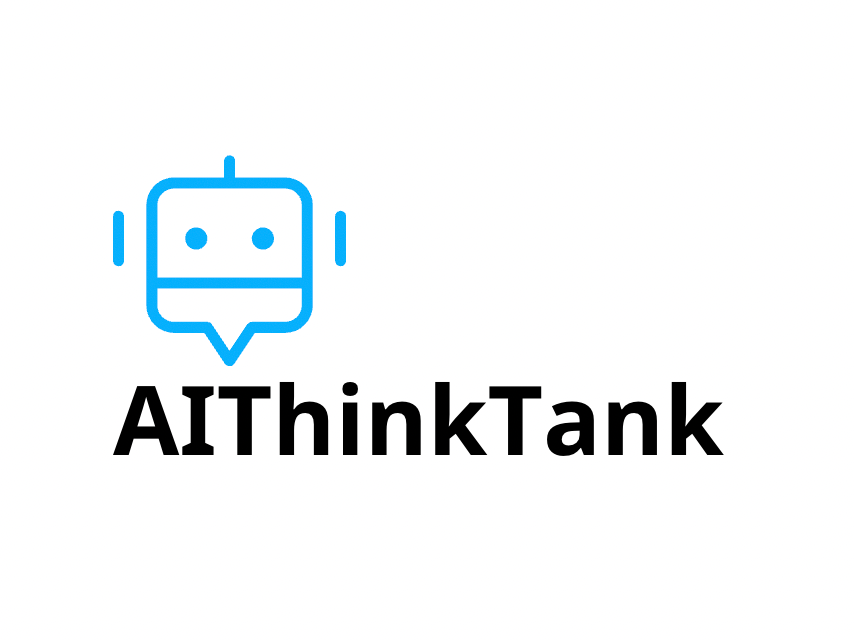
1. Machine Learning & Deep Learning
Key Models:
Linear & Logistic Regression: Basic models for regression and classification tasks.
Support Vector Machine (SVM): Classification and regression algorithm.
Random Forest & Decision Trees: Used for classification, regression, and feature selection.
K-Means & DBSCAN: Clustering algorithms for discovering patterns in data.
Neural Networks (NN): The backbone of deep learning, simulating how neurons in the brain process information.
Convolutional Neural Networks (CNN): Primarily for image processing tasks.
Recurrent Neural Networks (RNN) & Long Short-Term Memory (LSTM): Handle sequential data, e.g., time series or text.
Generative Adversarial Networks (GAN): Used for generating new data samples (images, videos, etc.).
Applications:
Healthcare: Image-based diagnostics (e.g., CNN for X-ray scans), disease prediction (e.g., logistic regression).
Finance: Fraud detection (e.g., decision trees, random forest), stock price prediction (e.g., RNN, LSTM).
E-commerce: Personalized recommendations (e.g., collaborative filtering, NN).
Natural Language Processing: Text classification and sentiment analysis (e.g., logistic regression, RNN).
2. Natural Language Processing (NLP)
Key Models:
Word Embeddings (Word2Vec, GloVe): Represent words as vectors, capturing semantic relationships.
Transformer: A deep learning model architecture for handling sequential data. Popularized by models like BERT and GPT.
BERT (Bidirectional Encoder Representations from Transformers): For natural language understanding (NLU) tasks.
GPT (Generative Pre-trained Transformer): For natural language generation (NLG) tasks.
Sequence-to-Sequence (Seq2Seq) Models: Used for tasks like machine translation, text summarization.
Attention Mechanism: Improves the capture of long-range dependencies in sequences.
Applications:
Chatbots & Virtual Assistants: GPT-3 for generating human-like responses.
Text Summarization: Seq2Seq models for summarizing documents.
Machine Translation: Transformer models are the backbone of services like Google Translate.
Sentiment Analysis: Word embeddings and Transformers are used to determine sentiment in text for market analysis.
3. Computer Vision (CV)
Key Models:
Convolutional Neural Networks (CNN): Specialized in image processing.
YOLO (You Only Look Once): Real-time object detection.
R-CNN (Region-based CNN), Faster R-CNN: High-precision object detection.
UNet: Image segmentation, particularly in medical imaging.
Generative Adversarial Networks (GAN): Image generation, such as DeepFake or artistic styles.
Vision Transformers (ViT): A transformer-based model for image processing, gradually replacing CNN in some tasks.
Applications:
Healthcare: Medical imaging analysis (e.g., UNet for tumour segmentation).
Autonomous Vehicles: Real-time object detection (e.g., YOLO for pedestrian detection).
Security & Surveillance: Object detection in surveillance video (e.g., Faster R-CNN).
Art & Creativity: GAN for generating new artworks or faces.
4. Reinforcement Learning (RL)
Key Models:
Q-Learning: A basic algorithm for learning policies in RL tasks.
Deep Reinforcement Learning (DRL): Combines deep learning with RL; e.g., Deep Q-Network (DQN).
Policy Gradient Methods: Proximal Policy Optimization (PPO), Asynchronous Advantage Actor-Critic (A3C).
AlphaGo / AlphaZero: RL models designed for mastering board games like Go and Chess.
Applications:
Robotics: AI systems in robotics use RL to learn complex tasks like walking, grasping, and navigation.
Autonomous Vehicles: RL is used to improve decision-making and navigation.
Game AI: DeepMind's AlphaGo for playing and winning board games.
Energy Management: Optimizing power grids or data center cooling systems using RL.
5. Generative Models
Key Models:
Generative Adversarial Networks (GAN): Used to generate new data (images, videos, etc.) that resemble the training data.
Variational Autoencoders (VAE): A generative model that learns the latent representation of data.
Diffusion Models (e.g., DDPM): Generate high-quality images by learning how to reverse noise in a data sample.
Autoregressive Models (e.g., PixelCNN): Generate images pixel-by-pixel, modelling the dependencies between pixels.
Applications:
Art & Design: GANs create new artworks and generate realistic faces (e.g., StyleGAN).
Video Game Design: Procedural generation of game content, such as new levels or characters.
Healthcare: VAE can be used for medical imaging data augmentation, helping with rare disease detection.
Text-to-Image Models: DALL·E and Stable Diffusion use text prompts to generate corresponding images.
6. Big Data & Cloud Computing
Key Technologies:
Hadoop: Distributed computing framework for handling big data.
Spark: Faster, in-memory big data processing framework.
Distributed Deep Learning (e.g., Horovod): For scaling deep learning across multiple machines.
Cloud Computing Platforms: AWS, Google Cloud, and Azure provide AI infrastructure for training and deploying models.
Applications:
Data Analysis: Processing large datasets for business analytics, customer insights, and predictive modelling.
Healthcare: Cloud platforms for storing and analyzing large medical datasets (e.g., patient records, medical images).
Finance: Real-time fraud detection and market prediction using big data and cloud computing.
Autonomous Systems: Cloud-based infrastructure for real-time processing in autonomous vehicles.
7. Multimodal Learning
Key Models:
CLIP (Contrastive Language-Image Pretraining): Learns joint representations of images and their descriptions.
DALL·E: Generates images from textual descriptions.
ALIGN (A Large-scale ImaGe and Noisy-text embedding model): Learns joint image-text representations.
Applications:
Cross-modal Retrieval: Finding relevant images based on text descriptions or vice versa.
Content Creation: DALL·E generates art, product designs, or even advertisements based on textual input.
E-commerce: Virtual try-ons and product recommendations that combine visual and textual information.
Healthcare: Multimodal learning in medical AI, combining image (MRI scans) and textual data (patient notes) for diagnosis.
Model History
1. Linear Regression / Logistic Regression (Pre-1980s)
Description: These are the simplest forms of machine learning models. Linear regression is used for predicting continuous outcomes, while logistic regression is used for binary classification tasks. Both models are foundational and help solve basic prediction and classification problems.
Applications: Used in predictive analytics, financial risk assessment, and medical diagnosis (e.g., predicting the probability of disease).
2. Support Vector Machines (SVM) (1990s)
Description: SVM is a supervised learning model used for classification and regression tasks. It works by finding the optimal hyperplane that maximizes the margin between different classes.
Applications: Used in face recognition, handwriting recognition, and bioinformatics for gene classification.
3. Random Forest & Decision Trees (1990s)
Description: Decision Trees are models that split data into subsets based on feature values, making decisions at each node. Random Forest is an ensemble method that builds multiple decision trees and merges their results to improve accuracy and robustness.
Applications: Used in fraud detection, medical diagnosis, and recommendation systems.
4. K-Means Clustering (Late 1990s)
Description: K-Means is an unsupervised learning algorithm that groups data into clusters by minimizing the distance between the data points and the center of each cluster.
Applications: Customer segmentation, image compression, market basket analysis.
5. Artificial Neural Networks (ANN) (1990s-2000s)
Description: ANNs simulate how neurons in the brain work, where each node is a neuron that applies a weight to its input. They are foundational to deep learning and can be used for a variety of tasks, such as regression, classification, and pattern recognition.
Applications: Used in speech recognition, character recognition, and time-series forecasting.
6. Recurrent Neural Networks (RNN) (1990s)
Description: RNNs are designed for sequence data (e.g., time series, language). They maintain a memory of previous inputs through loops in their architecture, allowing them to handle temporal dependencies.
Applications: Language modeling, sentiment analysis, and speech recognition.
7. Convolutional Neural Networks (CNN) (1998)
Description: CNNs are a type of deep learning model primarily used for image data. They apply convolutional filters to images to detect features like edges, textures, and shapes.
Applications: Image classification (e.g., facial recognition), object detection, and medical image analysis (e.g., tumour detection).
8. K-Means (1999)
Description: An unsupervised learning algorithm used to find clusters in data. It tries to partition data into k distinct clusters based on distance.
Applications: Customer segmentation, document classification, and data compression.
9. Support Vector Machines (SVM) (Late 1990s)
Description: A supervised learning model used for classification and regression. It tries to find the hyperplane that maximally separates data points from different classes.
Applications: Image classification, text categorization, and bioinformatics.
10. Long Short-Term Memory (LSTM) (1997)
Description: LSTM is a special kind of RNN that can learn long-term dependencies, making it suitable for tasks where the order of information matters over long sequences.
Applications: Language translation, speech recognition, time-series forecasting.
11. Autoencoders (2006)
Description: Autoencoders are neural networks designed to compress data into a lower-dimensional representation and then reconstruct the data. They are often used for dimensionality reduction and data denoising.
Applications: Image denoising, anomaly detection, and dimensionality reduction for large datasets.
12. Restricted Boltzmann Machines (RBM) (2007)
Description: A type of neural network that learns to reconstruct input data by modelling the underlying probability distribution of the input. It is often used in unsupervised learning tasks.
Applications: Feature learning, collaborative filtering, and dimensionality reduction.
13. Variational Autoencoder (VAE) (2013)
Description: A VAE is a generative model that learns the latent representation of the input data while incorporating probabilistic reasoning, allowing it to generate new, similar data points.
Applications: Image generation, data augmentation, anomaly detection in images and sensor data.
14. Deep Q-Network (DQN) (2013)
Description: A combination of Q-learning and deep learning, DQN is used in reinforcement learning (RL). It can handle complex decision-making tasks by learning policies from the environment.
Applications: Game AI (e.g., Atari games), robotic control, autonomous systems.
15. Generative Adversarial Networks (GAN) (2014)
Description: GAN consists of two neural networks: a generator and a discriminator. The generator creates fake data (e.g., images), while the discriminator tries to distinguish between real and fake data. The two networks compete, leading to the generation of increasingly realistic data.
Applications: Image synthesis (e.g., generating realistic human faces), video generation, style transfer, and data augmentation.
16. AlphaGo / AlphaZero (2015-2017)
Description: These are reinforcement learning systems designed by DeepMind. AlphaGo learned to play Go by analyzing expert games, while AlphaZero generalized the approach, learning to master multiple games (Go, Chess, Shogi) by self-play.
Applications: Mastery of complex board games, strategy optimization, and decision-making in autonomous systems.
17. Sequence-to-Sequence (Seq2Seq) Model (2014)
Description: A neural network model used for transforming one sequence into another sequence, such as translating a sentence from one language to another. It is a foundational architecture for tasks like language translation and text summarization.
Applications: Machine translation (e.g., Google Translate), text summarization, and speech recognition.
18. Transformer Model (2017)
Description: Transformers are designed to handle sequential data by using self-attention mechanisms to capture long-range dependencies. They have become the foundation for many state-of-the-art models in NLP.
Applications: Text translation, document summarization, text generation, and image captioning.
19. BERT (Bidirectional Encoder Representations from Transformers) (2018)
Description: BERT is a pre-trained language model that can be fine-tuned for various NLP tasks such as question answering and sentiment analysis. It captures bidirectional context, allowing it to understand the meaning of words based on all of the surrounding text.
Applications: Text classification, sentiment analysis, question-answering systems, and named entity recognition.
20. GPT (Generative Pretrained Transformer) (2018-2023)
Description: GPT models (from GPT-1 to GPT-4) are large-scale language models that use the transformer architecture to generate human-like text. GPT-3 and GPT-4 can perform a wide range of tasks with little or no task-specific training.
Applications: Text generation, chatbots, language translation, summarization, and creative writing.
21. Diffusion Models (2020)
Description: Diffusion models generate data by reversing a gradual noising process. These models produce high-quality images and have emerged as a leading method in generative AI.
Applications: High-resolution image synthesis, 3D object generation, and video generation.
22. CLIP (Contrastive Language-Image Pretraining) (2021)
Description: CLIP is a multimodal model that connects text and images by learning joint representations. It can understand and generate images based on text prompts.
Applications: Cross-modal retrieval, image captioning, text-to-image generation (in models like DALL·E).
23. DALL·E (2021)
Description: DALL·E is a transformer-based model that can generate images from textual descriptions. It demonstrates the ability to create highly detailed and contextually relevant images based on user prompts.
Applications: Text-to-image generation, creative content generation, and design automation.
24. Stable Diffusion (2022)
Description: Stable Diffusion is an advanced diffusion model for generating high-quality images. It offers a lightweight approach to creating complex images with fine details and realistic effects.
Applications: Art and design, text-to-image generation, 3D modelling, and video generation.
Summary of Core Technologies and Applications
Model/Technology | Primary Applications | Key Models |
Machine Learning | Predictive modelling, data analysis | SVM, Decision Trees, Random Forest |
Deep Learning | Image, speech recognition, fraud detection | CNN, RNN, LSTM, GAN |
NLP | Text generation, translation, sentiment analysis | Transformer, BERT, GPT, Seq2Seq |
Computer Vision | Image classification, object detection, medical imaging | CNN, YOLO, R-CNN, Vision Transformers |
Reinforcement Learning | Autonomous systems, robotics, games | DQN, PPO, AlphaGo, AlphaZero |
Generative Models | Art creation, synthetic data generation | GAN, VAE, Diffusion Models, PixelCNN |
Big Data & Cloud | Large-scale data processing, real-time decision-making | Hadoop, Spark, AWS, Google Cloud, Azure |
Multimodal Learning | Text-to-image generation, cross-modal search | CLIP, DALL·E, ALIGN |
Komentar